Estimating effect of multiple treatments
[1]:
import numpy as np
import pandas as pd
import logging
import dowhy
from dowhy import CausalModel
import dowhy.datasets
import econml
import warnings
warnings.filterwarnings('ignore')
[2]:
data = dowhy.datasets.linear_dataset(10, num_common_causes=4, num_samples=10000,
num_instruments=0, num_effect_modifiers=2,
num_treatments=2,
treatment_is_binary=False,
num_discrete_common_causes=2,
num_discrete_effect_modifiers=0,
one_hot_encode=False)
df=data['df']
df.head()
[2]:
X0 | X1 | W0 | W1 | W2 | W3 | v0 | v1 | y | |
---|---|---|---|---|---|---|---|---|---|
0 | -0.413136 | 1.588226 | 0.487770 | 0.290617 | 0 | 0 | 1.133998 | 4.769532 | 79.134513 |
1 | -1.134280 | -0.198177 | -1.796690 | 2.493473 | 3 | 1 | 11.873946 | 15.240956 | -22.448157 |
2 | -0.040000 | -0.259943 | 0.509664 | 2.970383 | 3 | 0 | 14.436375 | 21.720983 | 178.499781 |
3 | 0.428227 | -0.179278 | 1.130956 | -1.010133 | 0 | 3 | 3.065979 | 15.740535 | 200.564517 |
4 | 0.879490 | -0.668984 | -1.299954 | 0.571345 | 3 | 2 | 8.741953 | 19.404153 | 194.187850 |
[3]:
model = CausalModel(data=data["df"],
treatment=data["treatment_name"], outcome=data["outcome_name"],
graph=data["gml_graph"])
[4]:
model.view_model()
from IPython.display import Image, display
display(Image(filename="causal_model.png"))
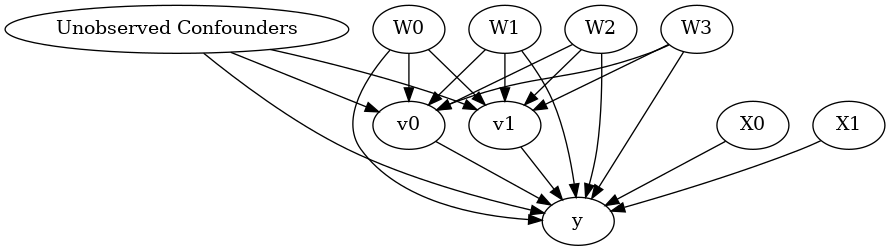
[5]:
identified_estimand= model.identify_effect(proceed_when_unidentifiable=True)
print(identified_estimand)
Estimand type: nonparametric-ate
### Estimand : 1
Estimand name: backdoor
Estimand expression:
d
─────────(Expectation(y|X1,W0,W2,W3,X0,W1))
d[v₀ v₁]
Estimand assumption 1, Unconfoundedness: If U→{v0,v1} and U→y then P(y|v0,v1,X1,W0,W2,W3,X0,W1,U) = P(y|v0,v1,X1,W0,W2,W3,X0,W1)
### Estimand : 2
Estimand name: iv
No such variable found!
### Estimand : 3
Estimand name: frontdoor
No such variable found!
Linear model
Let us first see an example for a linear model. The control_value and treatment_value can be provided as a tuple/list when the treatment is multi-dimensional.
The interpretation is change in y when v0 and v1 are changed from (0,0) to (1,1).
[6]:
linear_estimate = model.estimate_effect(identified_estimand,
method_name="backdoor.linear_regression",
control_value=(0,0),
treatment_value=(1,1),
method_params={'need_conditional_estimates': False})
print(linear_estimate)
*** Causal Estimate ***
## Identified estimand
Estimand type: nonparametric-ate
### Estimand : 1
Estimand name: backdoor
Estimand expression:
d
─────────(Expectation(y|X1,W0,W2,W3,X0,W1))
d[v₀ v₁]
Estimand assumption 1, Unconfoundedness: If U→{v0,v1} and U→y then P(y|v0,v1,X1,W0,W2,W3,X0,W1,U) = P(y|v0,v1,X1,W0,W2,W3,X0,W1)
## Realized estimand
b: y~v0+v1+X1+W0+W2+W3+X0+W1+v0*X1+v0*X0+v1*X1+v1*X0
Target units: ate
## Estimate
Mean value: 26.41126316018485
You can estimate conditional effects, based on effect modifiers.
[7]:
linear_estimate = model.estimate_effect(identified_estimand,
method_name="backdoor.linear_regression",
control_value=(0,0),
treatment_value=(1,1))
print(linear_estimate)
*** Causal Estimate ***
## Identified estimand
Estimand type: nonparametric-ate
### Estimand : 1
Estimand name: backdoor
Estimand expression:
d
─────────(Expectation(y|X1,W0,W2,W3,X0,W1))
d[v₀ v₁]
Estimand assumption 1, Unconfoundedness: If U→{v0,v1} and U→y then P(y|v0,v1,X1,W0,W2,W3,X0,W1,U) = P(y|v0,v1,X1,W0,W2,W3,X0,W1)
## Realized estimand
b: y~v0+v1+X1+W0+W2+W3+X0+W1+v0*X1+v0*X0+v1*X1+v1*X0
Target units: ate
## Estimate
Mean value: 26.41126316018485
### Conditional Estimates
__categorical__X1 __categorical__X0
(-3.537, -1.017] (-2.9979999999999998, 0.0335] -52.946620
(0.0335, 0.628] -36.698335
(0.628, 1.109] -28.405206
(1.109, 1.706] -17.981903
(1.706, 5.283] -2.357185
(-1.017, -0.424] (-2.9979999999999998, 0.0335] -19.631323
(0.0335, 0.628] -3.214915
(0.628, 1.109] 6.064157
(1.109, 1.706] 15.879803
(1.706, 5.283] 32.015991
(-0.424, 0.0749] (-2.9979999999999998, 0.0335] 0.264664
(0.0335, 0.628] 16.556340
(0.628, 1.109] 26.187937
(1.109, 1.706] 36.937134
(1.706, 5.283] 52.720748
(0.0749, 0.645] (-2.9979999999999998, 0.0335] 21.404275
(0.0335, 0.628] 37.196526
(0.628, 1.109] 46.502518
(1.109, 1.706] 56.370240
(1.706, 5.283] 72.695058
(0.645, 3.36] (-2.9979999999999998, 0.0335] 55.298602
(0.0335, 0.628] 70.148621
(0.628, 1.109] 79.236172
(1.109, 1.706] 89.107877
(1.706, 5.283] 107.081877
dtype: float64
More methods
You can also use methods from EconML or CausalML libraries that support multiple treatments. You can look at examples from the conditional effect notebook: https://microsoft.github.io/dowhy/example_notebooks/dowhy-conditional-treatment-effects.html
Propensity-based methods do not support multiple treatments currently.