Demo for the DoWhy causal API
We show a simple example of adding a causal extension to any dataframe.
[1]:
import dowhy.datasets
import dowhy.api
import numpy as np
import pandas as pd
from statsmodels.api import OLS
[2]:
data = dowhy.datasets.linear_dataset(beta=5,
num_common_causes=1,
num_instruments = 0,
num_samples=1000,
treatment_is_binary=True)
df = data['df']
df['y'] = df['y'] + np.random.normal(size=len(df)) # Adding noise to data. Without noise, the variance in Y|X, Z is zero, and mcmc fails.
#data['dot_graph'] = 'digraph { v ->y;X0-> v;X0-> y;}'
treatment= data["treatment_name"][0]
outcome = data["outcome_name"][0]
common_cause = data["common_causes_names"][0]
df
[2]:
W0 | v0 | y | |
---|---|---|---|
0 | 0.495676 | False | 0.114497 |
1 | 0.271500 | True | 6.111279 |
2 | 0.405087 | True | 4.467770 |
3 | 1.437909 | True | 10.856139 |
4 | 0.853315 | False | 5.036457 |
... | ... | ... | ... |
995 | 0.683802 | False | 0.712246 |
996 | -0.649195 | False | -3.760712 |
997 | 1.223760 | False | 4.286952 |
998 | 2.577242 | True | 11.527125 |
999 | 0.762801 | True | 6.146325 |
1000 rows × 3 columns
[3]:
# data['df'] is just a regular pandas.DataFrame
df.causal.do(x=treatment,
variable_types={treatment: 'b', outcome: 'c', common_cause: 'c'},
outcome=outcome,
common_causes=[common_cause],
proceed_when_unidentifiable=True).groupby(treatment).mean().plot(y=outcome, kind='bar')
[3]:
<AxesSubplot: xlabel='v0'>
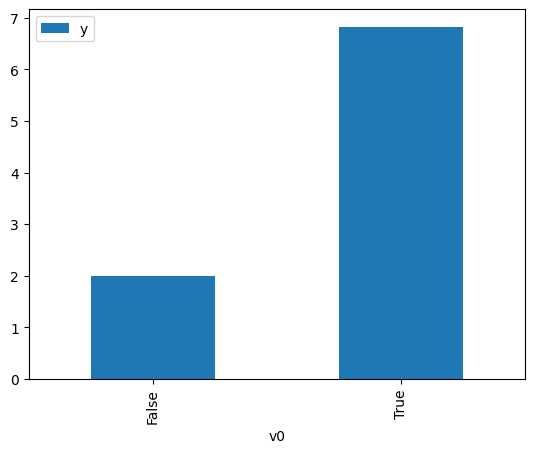
[4]:
df.causal.do(x={treatment: 1},
variable_types={treatment:'b', outcome: 'c', common_cause: 'c'},
outcome=outcome,
method='weighting',
common_causes=[common_cause],
proceed_when_unidentifiable=True).groupby(treatment).mean().plot(y=outcome, kind='bar')
[4]:
<AxesSubplot: xlabel='v0'>
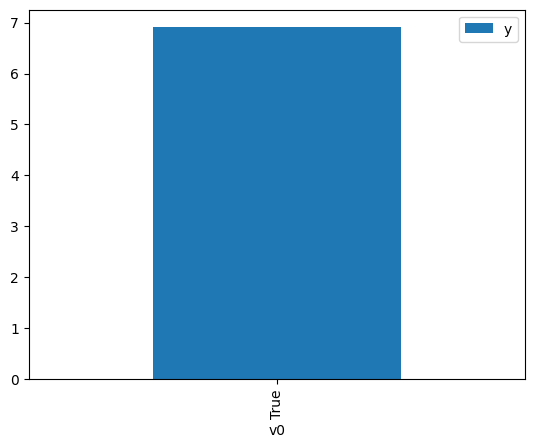
[5]:
cdf_1 = df.causal.do(x={treatment: 1},
variable_types={treatment: 'b', outcome: 'c', common_cause: 'c'},
outcome=outcome,
dot_graph=data['dot_graph'],
proceed_when_unidentifiable=True)
cdf_0 = df.causal.do(x={treatment: 0},
variable_types={treatment: 'b', outcome: 'c', common_cause: 'c'},
outcome=outcome,
dot_graph=data['dot_graph'],
proceed_when_unidentifiable=True)
[6]:
cdf_0
[6]:
W0 | v0 | y | propensity_score | weight | |
---|---|---|---|---|---|
0 | 0.962677 | False | 2.731163 | 0.488649 | 2.046457 |
1 | 0.963060 | False | 1.285152 | 0.488636 | 2.046512 |
2 | 1.333450 | False | 2.819011 | 0.476049 | 2.100623 |
3 | 1.113292 | False | 4.793211 | 0.483528 | 2.068131 |
4 | -0.076380 | False | 1.492980 | 0.523985 | 1.908450 |
... | ... | ... | ... | ... | ... |
995 | 0.495474 | False | 1.407595 | 0.504545 | 1.981984 |
996 | 0.093358 | False | 2.586551 | 0.518220 | 1.929683 |
997 | -0.649195 | False | -3.760712 | 0.543386 | 1.840313 |
998 | -0.313608 | False | -1.064077 | 0.532032 | 1.879586 |
999 | -1.104398 | False | -3.100139 | 0.558712 | 1.789830 |
1000 rows × 5 columns
[7]:
cdf_1
[7]:
W0 | v0 | y | propensity_score | weight | |
---|---|---|---|---|---|
0 | 1.800934 | True | 9.604396 | 0.539792 | 1.852566 |
1 | 0.908676 | True | 7.848732 | 0.509514 | 1.962655 |
2 | 1.486907 | True | 8.214336 | 0.529158 | 1.889796 |
3 | 2.860181 | True | 11.082506 | 0.575341 | 1.738098 |
4 | 0.605184 | True | 8.075637 | 0.499188 | 2.003253 |
... | ... | ... | ... | ... | ... |
995 | 0.762801 | True | 6.146325 | 0.504551 | 1.981959 |
996 | -1.252360 | True | 2.651436 | 0.436328 | 2.291852 |
997 | -0.702970 | True | 1.747041 | 0.454799 | 2.198774 |
998 | 1.964816 | True | 8.846990 | 0.545328 | 1.833759 |
999 | 1.906254 | True | 6.299404 | 0.543351 | 1.840431 |
1000 rows × 5 columns
Comparing the estimate to Linear Regression
First, estimating the effect using the causal data frame, and the 95% confidence interval.
[8]:
(cdf_1['y'] - cdf_0['y']).mean()
[8]:
$\displaystyle 4.70464995857471$
[9]:
1.96*(cdf_1['y'] - cdf_0['y']).std() / np.sqrt(len(df))
[9]:
$\displaystyle 0.22224585666512$
Comparing to the estimate from OLS.
[10]:
model = OLS(np.asarray(df[outcome]), np.asarray(df[[common_cause, treatment]], dtype=np.float64))
result = model.fit()
result.summary()
[10]:
Dep. Variable: | y | R-squared (uncentered): | 0.968 |
---|---|---|---|
Model: | OLS | Adj. R-squared (uncentered): | 0.968 |
Method: | Least Squares | F-statistic: | 1.518e+04 |
Date: | Mon, 27 Mar 2023 | Prob (F-statistic): | 0.00 |
Time: | 12:34:22 | Log-Likelihood: | -1439.3 |
No. Observations: | 1000 | AIC: | 2883. |
Df Residuals: | 998 | BIC: | 2892. |
Df Model: | 2 | ||
Covariance Type: | nonrobust |
coef | std err | t | P>|t| | [0.025 | 0.975] | |
---|---|---|---|---|---|---|
x1 | 2.3676 | 0.029 | 82.056 | 0.000 | 2.311 | 2.424 |
x2 | 4.9885 | 0.052 | 96.714 | 0.000 | 4.887 | 5.090 |
Omnibus: | 2.708 | Durbin-Watson: | 1.914 |
---|---|---|---|
Prob(Omnibus): | 0.258 | Jarque-Bera (JB): | 2.655 |
Skew: | -0.079 | Prob(JB): | 0.265 |
Kurtosis: | 3.197 | Cond. No. | 2.21 |
Notes:
[1] R² is computed without centering (uncentered) since the model does not contain a constant.
[2] Standard Errors assume that the covariance matrix of the errors is correctly specified.